6.12 EMP_network_analysis
The EMP_multi_analysis module aims to construct network relationships between features to analyze their interactions and identify key feature nodes. This module not only assesses the relationships within single-omics features but also further explores the network interactions between different omics, offering a more comprehensive systems biology perspective.
6.12.1 single-omics network
🏷️Example:
1.Network between differential genus taxa and coldata
Note:
Parameter
Parameter
coldata_to_assay
could inlcude the interesting coldata into the network.MAE |>
EMP_assay_extract('taxonomy') |>
EMP_collapse(estimate_group = 'Genus',collapse_by = 'row') |>
EMP_diff_analysis(method='wilcox.test、', estimate_group = 'Group') |>
EMP_filter(feature_condition = pvalue<0.05) |>
EMP_network_analysis(coldata_to_assay = c('BMI','PHQ9','GAD7'))
2.Visualization of the node importance.
MAE |>
EMP_assay_extract('taxonomy') |>
EMP_collapse(estimate_group = 'Genus',collapse_by = 'row') |>
EMP_diff_analysis(method='wilcox.test', estimate_group = 'Group') |>
EMP_filter(feature_condition = pvalue<0.05) |>
EMP_network_analysis(coldata_to_assay = c('BMI','PHQ9','GAD7')) |>
EMP_network_plot(show='node')
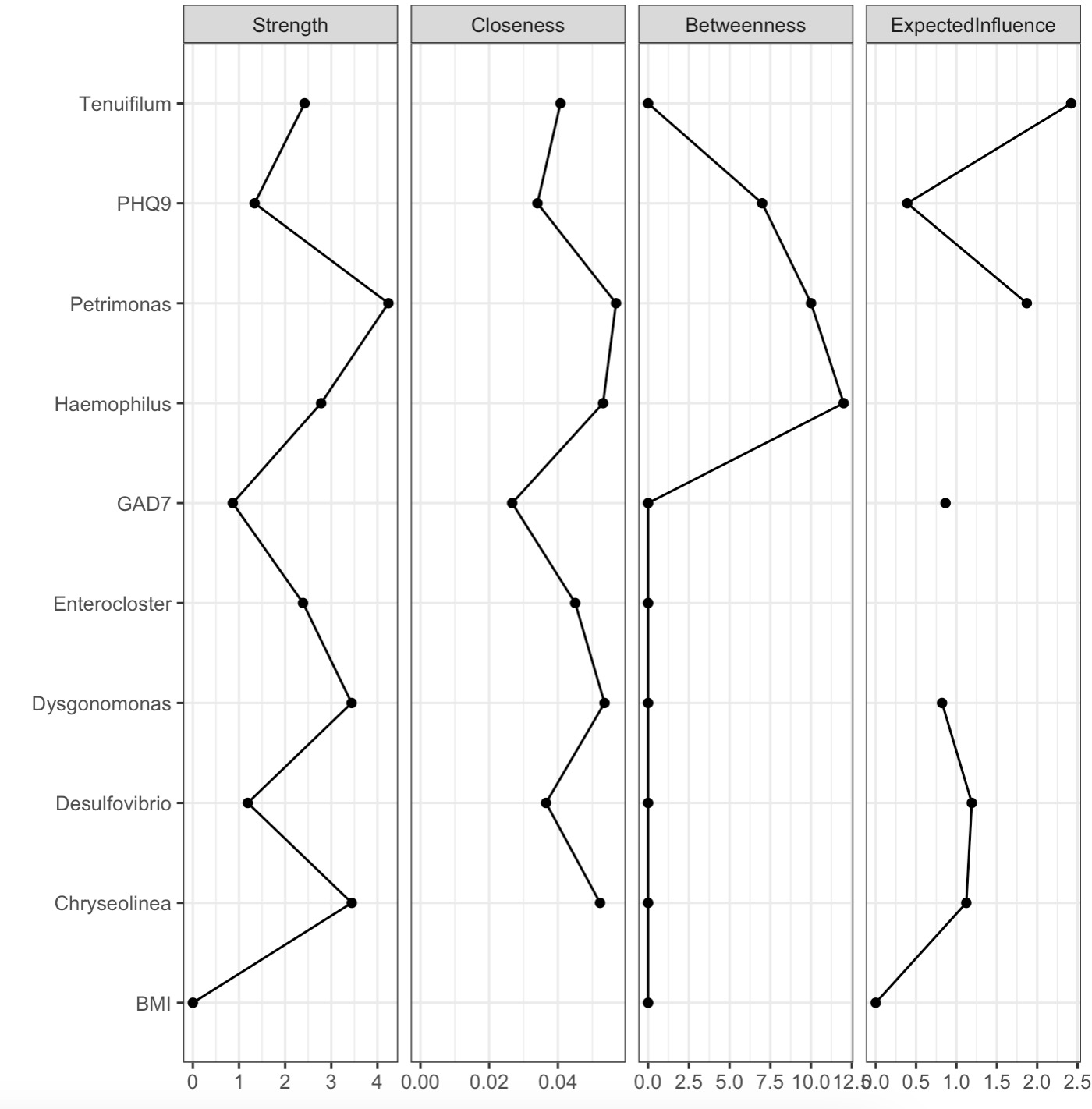
3.Filter the two most important taxa according to the network result
MAE |>
EMP_assay_extract('taxonomy') |>
EMP_collapse(estimate_group = 'Genus',collapse_by = 'row') |>
EMP_diff_analysis(method='wilcox.test', estimate_group = 'Group') |>
EMP_filter(feature_condition = pvalue<0.05) |>
EMP_network_analysis(coldata_to_assay = c('BMI','PHQ9','GAD7')) |>
EMP_filter(feature_condition = top_detect(Betweenness,2))
6.12.2 Multi-omics network
🏷️Example:
1.Network between microbiome and metabolite
Note:
The parameter
The parameter
threshold
can be used to select edges that are statistically significant or edges with adjusted p-values. (Default: threshold='sig'
)k1 <- MAE |>
EMP_assay_extract('taxonomy') |>
EMP_collapse(estimate_group = 'Genus',collapse_by = 'row') |>
EMP_diff_analysis(method='wilcox.test', estimate_group = 'Group') |>
EMP_filter(feature_condition = pvalue<0.05)
k2 <- MAE |>
EMP_collapse(experiment = 'untarget_metabol',na_string=c('NA','null','','-'),
estimate_group = 'MS2kegg',method = 'sum',collapse_by = 'row') |>
EMP_diff_analysis(method='DESeq2', .formula = ~Group) |>
EMP_filter(feature_condition = pvalue<0.05)
(k1 + k2 ) |>
EMP_network_analysis(threshold='sig')
2.Network visualization
Note:
①Paramter
②The parameter
③More paramters in the function qgraph.
①Paramter
node_info
could add more info to the node according to their rowdata.②The parameter
threshold
here, unlike the one in EMP_network_analysis
, specifies the minimum absolute value of the coefficient for edges to be displayed.③More paramters in the function qgraph.
(k1 + k2 ) |>
EMP_network_analysis() |>
EMP_network_plot(show = 'net',layout = 'spring',
shape='diamond',
edge.labels=TRUE,edge.label.cex=0.4,
vsize = 5,threshold = 0,
node_info = c('Phylum','MS2class'),
legend.cex=0.3,label.cex = 1,label.prop = 0.9,font=2)